AI called IKARUS identifies cancer cells.
- ACS BCP
- Jun 11, 2022
- 4 min read
With recent advancements with respect to the big C, we’re constantly learning and identifying new methods to fight it. The major backtrack though? Affecting only the cancer cells and not the regular cells in our body. How does a cure set them apart? Cue ‘IKARUS’, a new machine learning algorithm. The AI program has found a gene signature that is characteristic of tumours according to the team led by MDC bioinformatician Altuna Akalin in the journal Genome Biology.
Human beings are no match for artificial intelligence (AI) when it comes to identifying patterns in mountains of data. To reliably distinguish the cancer cells from the healthy cells, ‘IKARUS’ finds a pattern in tumour cells that is common to different types of cancer, consisting of a characteristic combination of genes.
Machine learning is the process of an algorithm learning how to answer problems on its own using training data. It accomplishes this by looking for patterns in the data that will assist it in solving the problem and thus generalise what it has learned after the training phase in order to evaluate unknown data.
The team used data from lung and colorectal cancer cells as their training data before applying it to data sets of other kinds of tumors.
In the training phase, Ikarus needed to compile a list of distinctive genes, which it then used to sort the cells into categories. Ikarus used two lists in the end: one for cancer genes and another for genes from other cells. Following the learning phase, the algorithm was able to reliably differentiate between healthy and malignant cells in tissue samples from patients with liver cancer and neuroblastoma. Even the research group was startled by how high its success rate was. They weren't expecting to find a shared characteristic that so clearly classified the tumour cells of various cancers. They intend to test ikarus on additional cancers in order to make it a viable tool for cancer diagnosis.
The project is expected to move beyond categorising cells as "healthy" or "cancerous." Ikarus has already proved that this approach can differentiate other types (and particular subtypes) of cells from tumour cells in its preliminary tests."We want to make the approach more comprehensive,” says Akalin, "developing it further so that it can distinguish between all possible cell types in a biopsy.”
Pathologists in hospitals typically only analyse tumour tissue samples under a microscope to identify the various cell types. It's a strenuous and timeconsuming task. With ikarus, this stage could be completely automated in the future. Akalin further points out that the data could be utilised to derive conclusions about the tumor's immediate environment. As the composition of malignant cells and the microenvironment typically determines whether or not a treatment or drug will be beneficial, doctors may be able to determine the optimal treatment option more accurately. Furthermore, AI may be effective in the development of new drugs. "Ikarus lets us identify genes that are potential drivers of cancer," Akalin says. These molecular structures can then be potentially targeted with novel therapeutic agents.
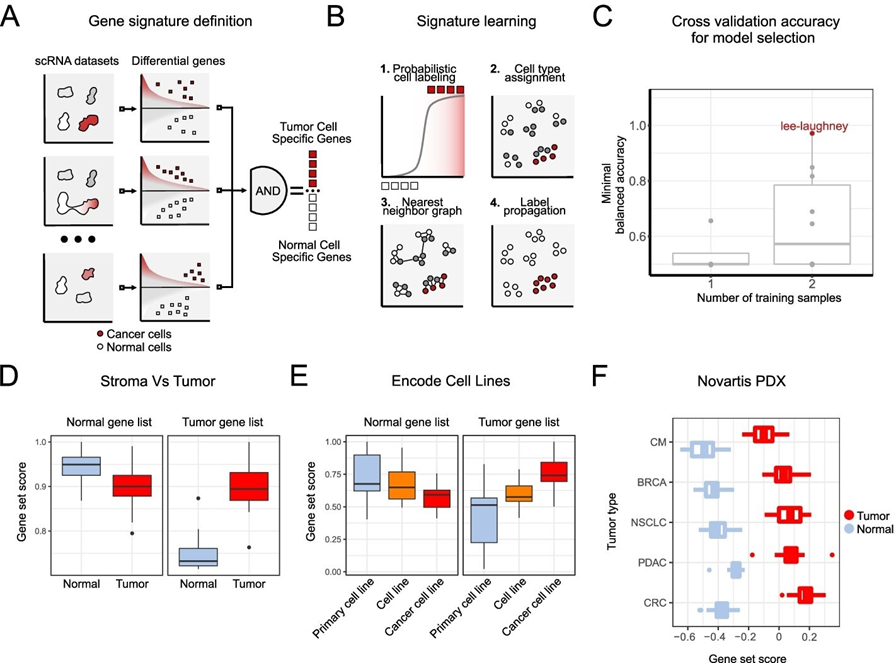
INTEGRATION OF MULTIPLE DATASETS ENABLES ROBUST EXTRACTION OF INFORMATIVE GENE SETS.
A, B - IKARUS WORKFLOW. IKARUS IS A TWO-STEP PROCEDURE FOR CLASSIFYING CELLS. IN
THE FIRST STEP, INTEGRATION OF MULTIPLE EXPERT LABELED DATASETS ENABLES THE
EXTRACTION OF ROBUST GENE MARKERS. THE GENE MARKERS ARE THEN USED IN A
COMPOSITE CLASSIFIER CONSISTING OF LOGISTIC REGRESSION AND NETWORK PROPAGATION.
C - COMPARISON OF CROSS VALIDATION ACCURACY FOR SIGNATURE DERIVATION AND MODEL
SELECTION. MINIMAL BALANCED ACCURACY ON THE VALIDATION SET WAS CHOSEN AS THE
METRIC OF CHOICE (I.E., WORSE PERFORMANCE ON THE TEST SET). MODELS TRAINED ON JUST
ONE DATASET ACHIEVED LOWER BALANCED ACCURACY THAN MODELS TRAINED ON TWO DATASETS (P VALUE GIVEN BY THE TWO SIDED WILCOXON TEST IS 0.063). THE COMBINATION OF COLORECTAL CANCER FROM LEE ET AL. AND LUNG CANCER FROM LAUGHNEY ET AL.
ACHIEVED THE HIGHEST MINIMAL BALANCED ACCURACY OF 0.97.
D - COMPARISON OF GENE SIGNATURE SCORES IN LASER MICRODISSECTED GASTRIC CANCER
DATA. THE NORMAL GENE LIST SHOWS LOWER SIGNATURE SCORES IN CANCER SAMPLES (P
VALUE 0.052, N = 8, MOOD'S MEDIAN TEST), WHEN COMPARED TO THE CANCER-ASSOCIATED NORMAL TISSUE. THE TUMOR GENE SIGNATURE IS SIGNIFICANTLY HIGHER FOR CANCER SAMPLES THAN THE NORMAL TISSUE (P VALUE 0.003, N = 8, MOOD'S MEDIAN TEST).
E - PRIMARY CELLS AND CANCER CELL LINES HAVE SIGNIFICANTLY DIFFERENT GENE
SIGNATURE DISTRIBUTIONS. THE NORMAL-CELL GENE SIGNATURE SHOWS A GRADUAL
REDUCTION IN GENE SIGNATURE SCORE DISTRIBUTION WHEN COMPARED IN PRIMARY CELLS,
CELL LINES, AND TUMOR CELL LINES. THE GENE SIGNATURE SHOWS THE COMPLETE OPPOSITE
EFFECT. CANCER CELL LINES HAVE THE HIGHER GENE SIGNATURE SCORE DISTRIBUTION,
FOLLOWED BY CELL LINES, AND PRIMARY CELLS. DISTRIBUTIONS WERE COMPARED USING PAIRWISE WILCOXON TESTS WITH BH-FDR CORRECTION. ALL ADJUSTED P VALUES WERE LOWER THAN 0.01.
F- PATIENT-DERIVED XENOGRAFTS (PDX) SHOW SIGNIFICANTLY HIGHER TUMOR GENE
SIGNATURE SCORE, THAN THE NORMAL GENE SIGNATURE SCORE. THE SAME PATTERN IS
OBSERVED IN MULTIPLE CANCER TYPES. NORMAL AND TUMOR SIGNATURE DISTRIBUTIONS
WERE COMPARED USING WILCOXON TESTS, FOR EACH CANCER TYPE, FOLLOWED BY BH-FDR
CORRECTION. ALL ADJUSTED P VALUES WERE LOWER THAN 0.01. CREDIT: GENOME BIOLOGY
(2022). DOI: 10.1186/S13059‐022‐02683‐1
References:
Altuna Akalin et al, Identifying tumor cells at the single-cell level using machine learning, Genome Biology (2022).
Comments